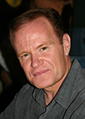
Tom Chittenden
President
Complex Biological Systems Alliance
USA
Biography
Thomas W. Chittenden is a Theoretical Systems Biologist and Founder of the Complex Biological Systems Alliance, a global scientific think tank dedicated to furthering scientific understanding of biological complexity and the nature and origins of human disease. Tom serves as a Senior Biostatistician and Mathematical Biologist for the Research Computing Group at the Harvard Medical School and a Scientific Advisor for the Pathway Genomics Corporation. He is a Research Fellow at the Vertex Society, an Associate Member of the International Society for Philosophical Enquiry, and a Member of the New England Complex Systems Institute. Tom holds a PhD in Molecular Cell Biology and Biotechnology from Virginia Tech and a DPhil in Computational Biology and Bioinformatics from the University of Oxford. His multidisciplinary postdoctoral training includes experimental investigations in Molecular and Cellular Cardiology at the Dartmouth Medical School and Integrative Functional Genomics at the Dana-Farber Cancer Institute and the Harvard School of Public Health.
Research Interest
A major focus of Tom’s research involves development and application of integrated systems biology models to investigate evolutionary factors of human disease. His overall objective is to understand how genetic variation and somatic mutation regulate aberrant gene activity and subsequent disease biology. To this end, Tom spent a year as a Visiting Research Scientist in the Department of Statistics at the University of Oxford, where he formulated a general strategy for constructing prediction models by integrating a priori biological knowledge with multiple types of high-throughput genomic data. This approach improves performance of established classification methods via enhanced semantic interoperability for mapping between multiple biomedical ontologies and the subsequent identification of genes more highly predictive of disease etiology.